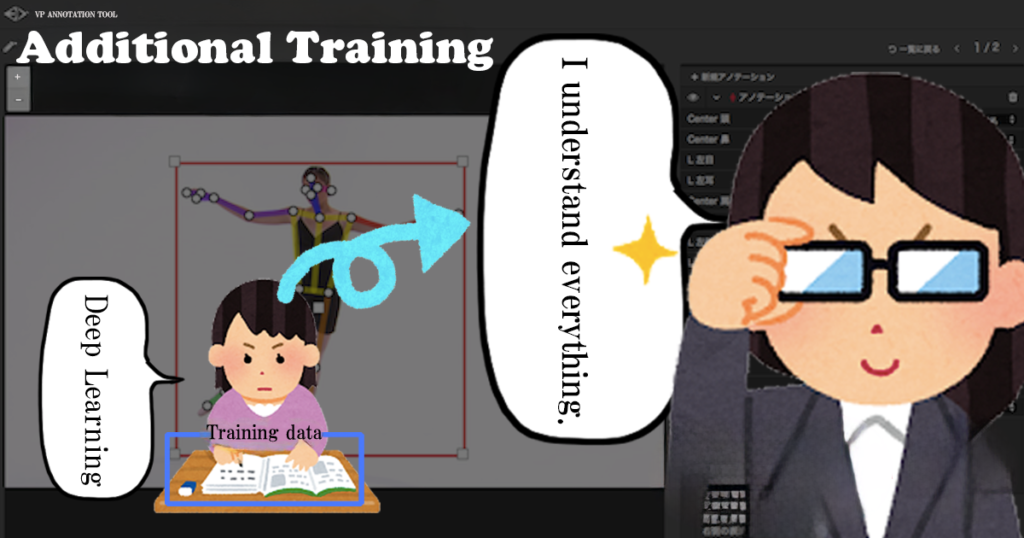
Hello, everyone. We are back again with another interesting update.
Presently, we are offering ‘VisionPose’ a system that employs AI(Deep Learning) and utilizes a webcam to detect human skeletal structures, showcasing the power of technology in enhancing various applications.
Anyway, do you have a question like this?
What if the skeleton detection is successful but the accuracy is poor?
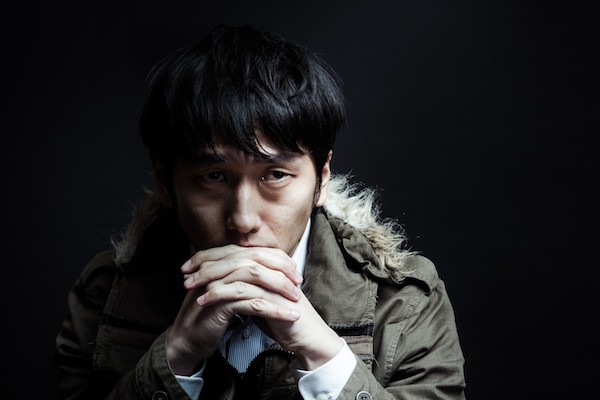
In general, when the accuracy of a camera with a depth sensor is low, there are often limited options for improvement, and the main solution is to change the environment.
In fact, our company has developed a system called Annotation Tool to create training data for specific movements.
As a result, we’ve gained the capability for additional training and the answer to that question might be here.
So, this time, we have conducted interviews with our developers. We will try to summarize the aspects of additional training with VisionPose as clearly as possible.
What is VisionPose?
Before diving into the main topic, let’s talk about ‘VisionPose,’ a system that uses Deep Learning and a webcam to detect the human skeleton.
Development of this domestic product began in response to the incident of Kinect being discontinued in October 2017. Currently, we receive inquiries from various fields such as research purposes, sports, and more.
Lately, we’ve been receiving many inquiries about using it for motion capture in MMD(MikuMikuDance). It seems to be a popular use case recently.
If you’re wondering what VisionPose is in the first place, please check it out here.
What is Additional Training?
Now that you understand what VisionPose is, let’s finally dive into today’s main topic.
VisionPose uses the information on ‘This is how the human skeleton looks like!’ to detect the skeleton.
However, since humans can assume various postures, it can be challenging to manually specify the location of the skeleton in millimeters for each movement.
Instead of training everything, we let VisionPose learn only the basics, and then it figures out the rest on its own.
As training materials, we use photo data containing correct skeletal coordinates for various postures (referred to as training data).
That’s a bit like a textbook with answers attached for studying the human skeleton.
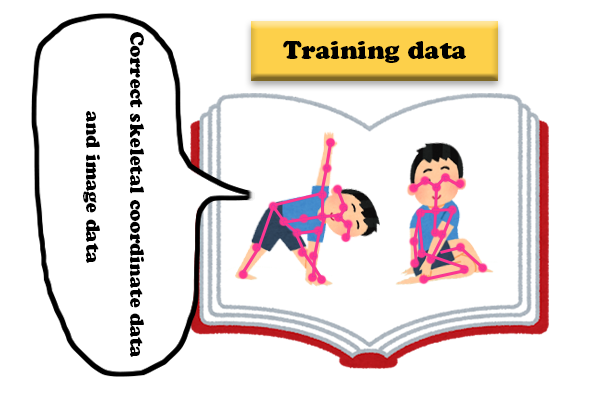
Once VisionPose is given this reference book, it works through problems based on its past experiences, checks the answers, and corrects it’s mistakes. This process is called Learning.
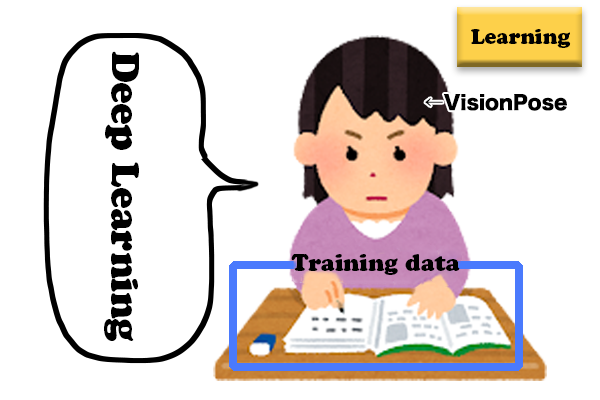
As a result, from numerous images, AI (Deep Learning) learns the rules of the human skeleton. The data trained using this training data is called Trained Tata.
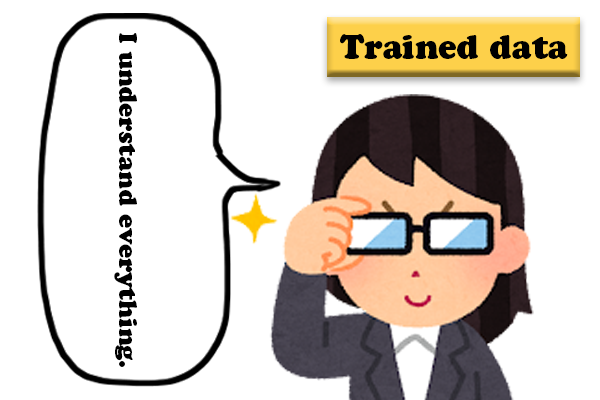
Once VisionPose has been trained, it can utilize the information it has learned from the provided training data to detect the human skeleton even in poses that were not included in the training data.
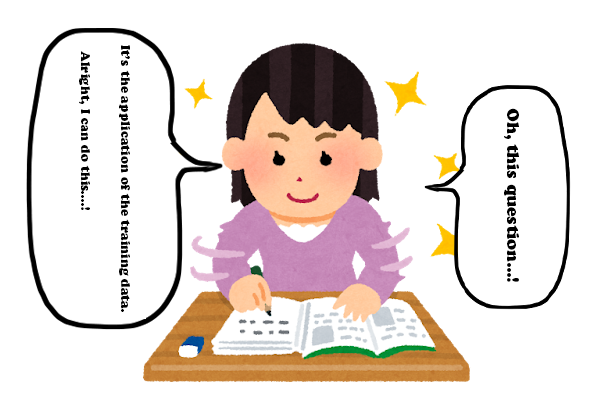
However, if the accuracy of the detected skeleton using this trained data is poor, it needs to be re-trained once again.
The act of training it again in this context is called Additional Training.
What changed with the ability for Additional Training?
Until the previous alpha version, additional training was not possible. Therefore, if the accuracy of the detected skeleton in the once-created trained data was poor, it was necessary to replace it with a different trained data.
However, in this latest version, Additional Training has become possible.
Therefore, if there is a difficult-to-detect movement in the initial skeleton detection by VisionPose, a cycle of further refinement can be conducted (learning → recognition → correction of recognition issues using the Annotation Tool → learning → recognition…). This allows for the continuous and efficient evolution of VisionPose (trained data) without unnecessary replacement.
Note: Additional training is an optional service. There are conditions for its implementation.
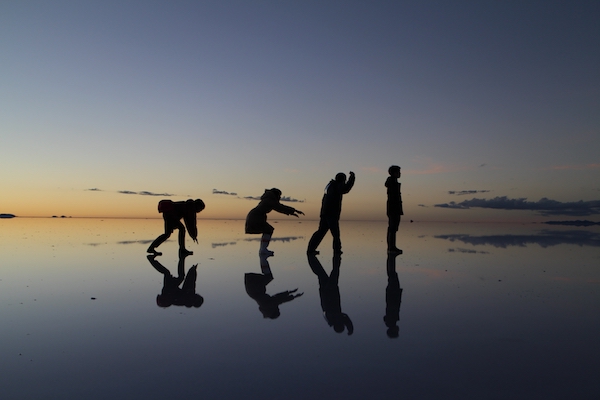
I think it might be a bit confusing, so let me simplify it. With the ability for additional training now, you can adjust the accuracy to fit our needs and further refine and improve it!
If there is a need to improve the accuracy of a specific movement, the process would involve working together with our company to undergo additional training and fine-tune the accuracy.
We will certainly provide maximum support!
What is Annotation tool?
An annotation tool is an optional tool in VisionPose, designed for creating training data for specific movements.
Various photos containing correct skeletal coordinates in different postures (referred to as training data). It’s like a reference book with answers for studying the human skeleton. As mentioned earlier, to enable additional training for VisionPose, this training data is required.
You can indeed commission another company to create the training data. However, if you rely on a different company each time for additional training, it could be challenging to coordinate, and there may be limitations in accommodating flexible customer requests.
For this reason, we developed our Annotation Tool in-house. Additionally, it’s a general characteristic in AI (machine learning) that improvement in accuracy is not guaranteed with every iteration of learning. After a certain point, there is a tendency for progress to plateau, and further enhancements become more challenging.
In the diagram below, the vertical axis represents the difference from the correct value. As the difference approaches zero, the accuracy improves. Therefore, learning is concluded when further reduction in the difference becomes difficult.
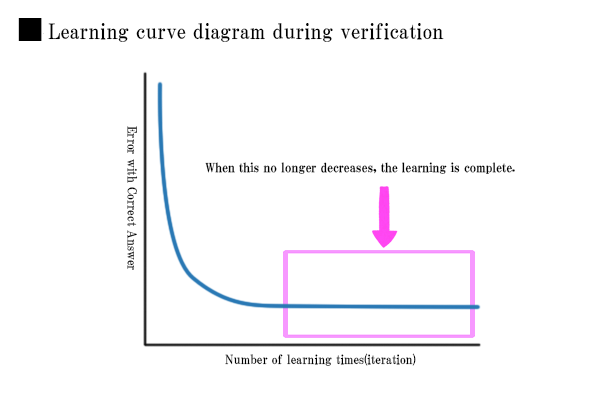
Therefore, it is necessary to identify the point where the accuracy is at its best. (In fact, this tuning part is often the most demanding and requires a sense of expertise.)
The main function of the annotation tool is precisely the editing of joint points.
Examples of use cases include the following:
- To detect the skeleton using VisionPose but having difficulty capturing the bones.
- To add joint points not present in the default VisionPose (consultation required).
Currently, VisionPose can detect a maximum of 30 joint points. If you wish to increase the limit, a consultation is required.
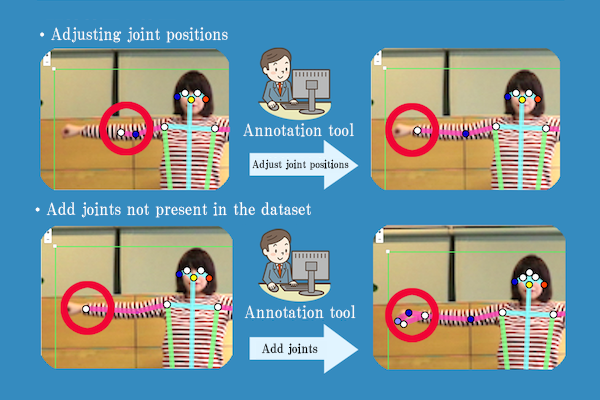
How to operate the Annotation Tool?
The creation of training data involves providing you with the annotation tool environment prepared by our company and perform the tasks on your end.
The operation is very simple! You will perform easy operations by just using the mouse. Let’s look at the examples below:
In the annotation tool, first, load the image and select the area where a person is present.
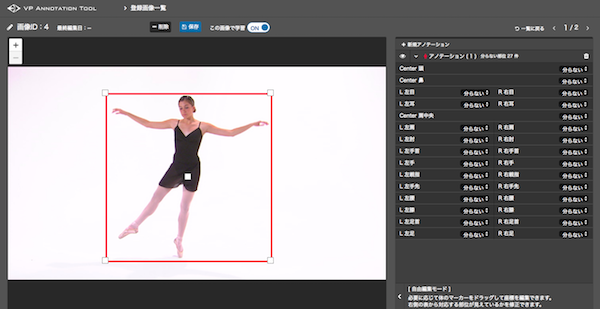
Then place points for each joint, aligning them with the person in the image.
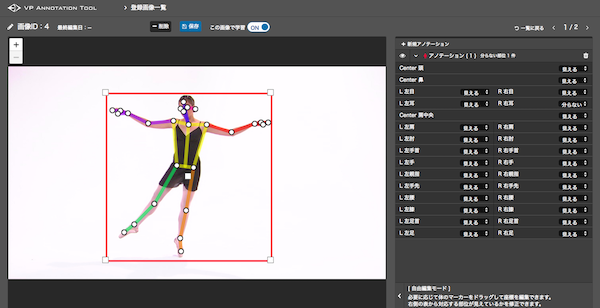
The positions of each body part and the points on the image are then reflected.
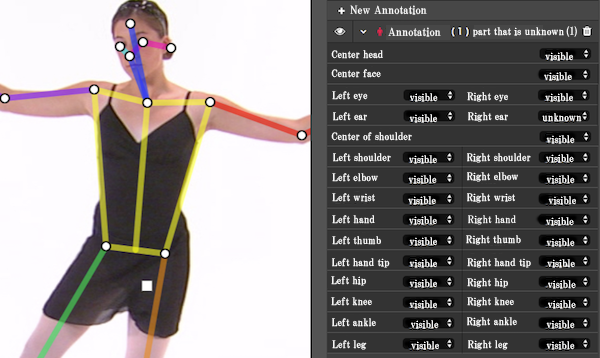
Once you confirm that the points are correctly positioned for each joint like this, save it, and one set of training data is complete!
Repeat this process many times.
What happens if you conduct Additional Training?
We conducted a test using the training data created with the annotation tool and the trained data obtained through additional training. It is evident that the detection works better after additional training compared to before.
Conclusion
I hope my explanation about Additional Training and VisionPose was clear and comprehensible.
If the accuracy was poor with VisionPose, you can use the annotation tool to create training data, adjust accuracy through additional training, and further refine the results.
The general flow of additional training is as follows:
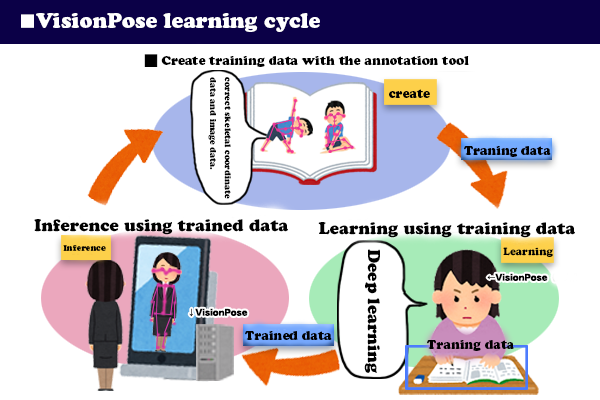
- Create training data with annotation tool
- Train VisionPose (pre-trained data) using the training data.
- Perform inference using VisionPose (pre-trained data). Confirm whether the skeleton is detected accurately.
- Repeat steps 1 to 3 until the accuracy improves.
Inference: Here, it refers to detecting the human skeleton using VisionPose.
VisionPose was initially released as an alpha version at the end of March 2018, mainly for early-adopting companies. We have been evolving through feedback and continuous improvements.
Currently, inquiries are high, and we are initially limiting access to corporate clients. If you would like more detailed information, please feel free to contact us.
If you would like to know more details about VisionPose, please inquire us here!
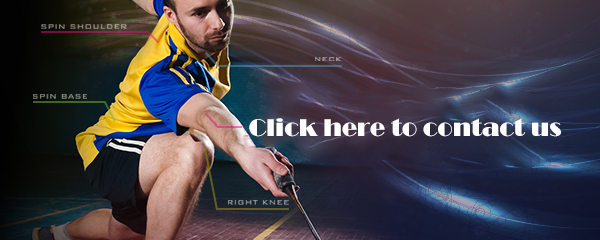
Additionally, you can also find us on Twitter, Facebook, Website & Linkedin.